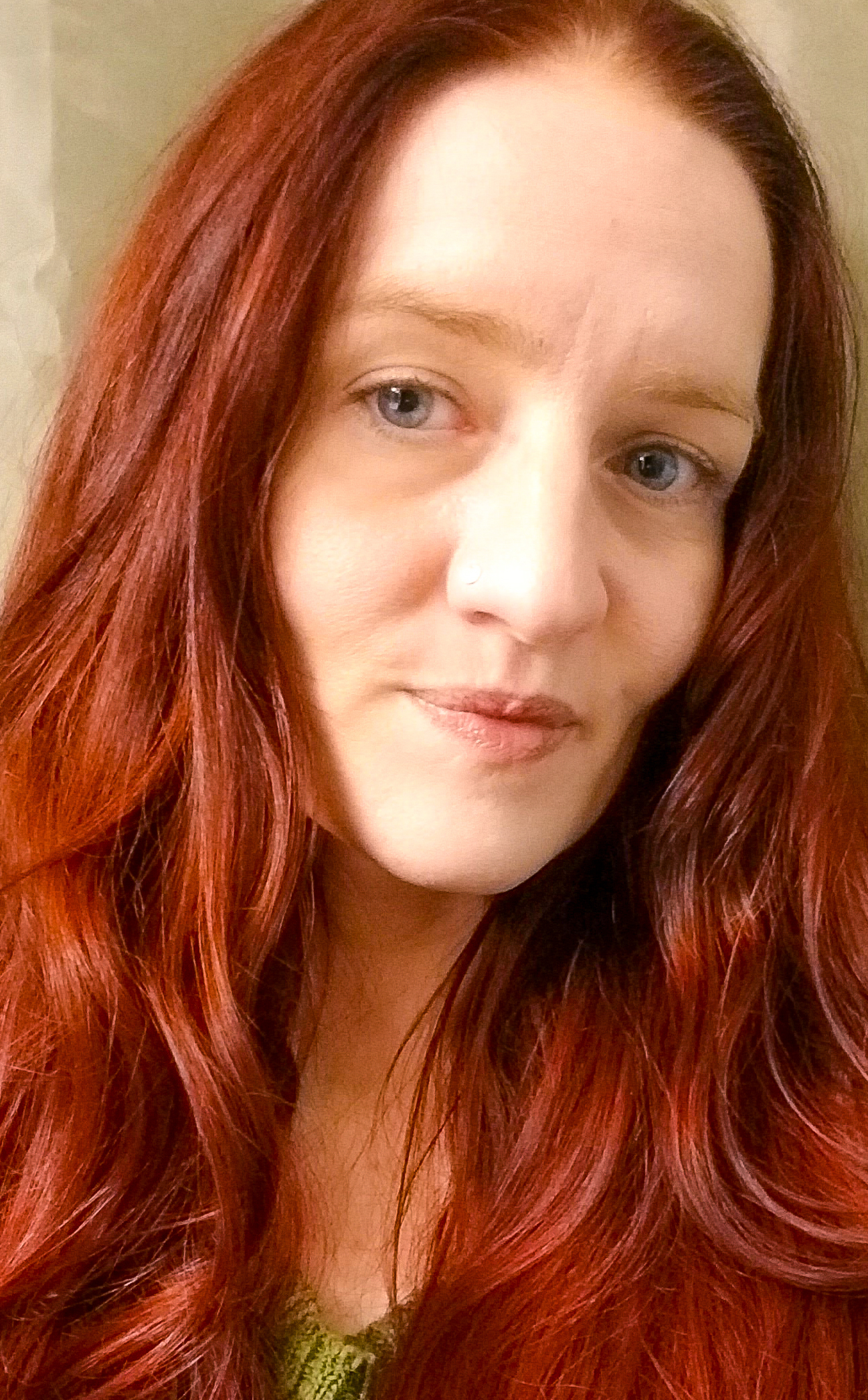
Mandy Hathaway
Because of its simplicity, this article is not meant to be the whole of your learning experience and educational links will be provided throughout so you can learn more.
Television, movies, books, everywhere you turn these days the discussion and stories often center around Artificial Intelligence (AI). Because of the media attention and our vast imaginations, understanding what is real and what is science-fiction can be a challenge. What is AI really like, and what is fantasy, at least for now? This short series will explore that and much more.
In this part of the series, we’re going to explore the risks associated with AI development. From lost jobs to biased systems, we’ll explore the realistic ethical and social risks that AI poses. For more information about how AI works, check out the first part of this series here, and to explore current AI applications and uses, check out the second part of this series here.
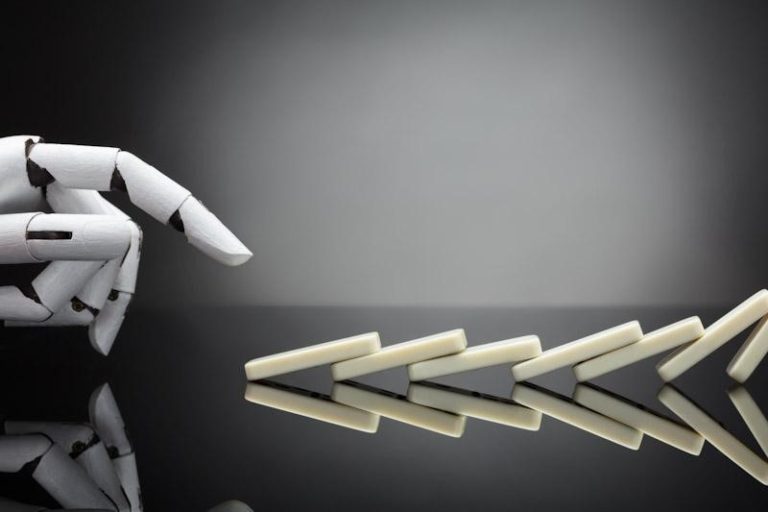
-Some Ethical Concerns
There can be little argument that AI, and technology in general, make some people nervous. It’s an increasingly common theme in movies and books. People worry about losing their jobs, getting hacked and about a computer uprising. But what’s the truth behind these fears? What are the real risks and how can we as a society prepare and manage the inevitable changes to come?
As discussed in part one of this series, AI is progressing; however, we’re still a significant distance from self-aware, sentient robots or computers. Right now, we have a wide variety of AI systems working in very particular areas with the potential to impact our lives and societies.
Privacy and Safety:
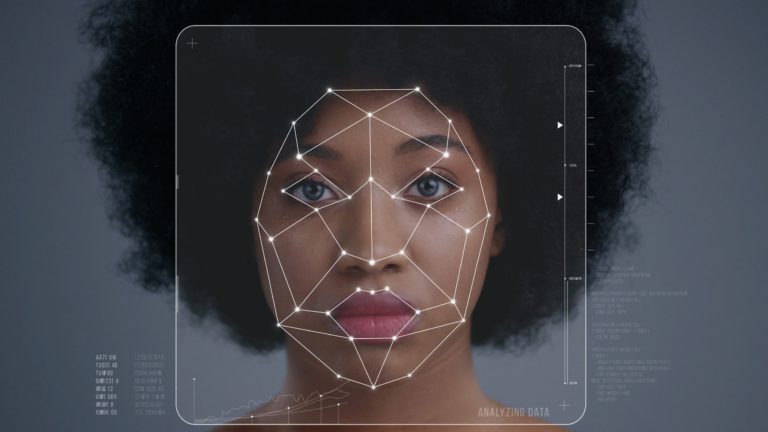
One of the most common causes of AI and technology related anxiety is the concern that they are invading our privacy and potentially exposing our personal (and sometimes intimate) details to strangers. Facial recognition software, if used properly, could potentially improve security. Still, many people are incredibly uncomfortable with having their location monitored and worry about the safety and reliability of these systems.
There is concern about the government and commercial businesses being able to monitor our location and travel. Even when used appropriately, people’s private information is made vulnerable for increased profitability on the part of businesses.
Even those who stay home and avoid technologies that monitor their location or use facial recognition do not find that their privacy remains intact. A large percentage of online companies, including the likes of Amazon, Facebook and Google, track your online behavior, even when you aren’t using their sites. They use the data they gather to increase the personalization of marketing and many collect and sell valuable databases of customer information.
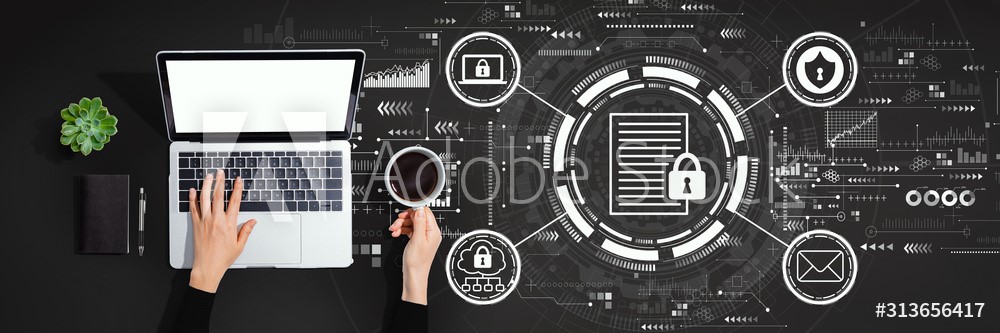
It isn’t just our location, shopping or banking information that is at risk. With the expansion of online data storage and access, more sensitive information like medical records can be put at risk.
In addition to what seems like constant data breaches in the news, law enforcement has used online ancestry sites—the kind that uses DNA to track family trees—to identify offenders in unsolved crimes. Some people argue that if you have nothing to hide, you have nothing to worry about, but many others deeply value the right to personal privacy.
Job Losses:
As if being worried about privacy and personal information wasn’t enough, many people are concerned about the automation of jobs. As things like automated stores and restaurants become a reality, there will likely be a loss of human jobs associated with the progress of AI. Some companies offer additional training and educational options to help prepare their workforce for impending changes. Many workers, especially older employees and those with limited education, reasonably fear the transition to automation in the workplace.
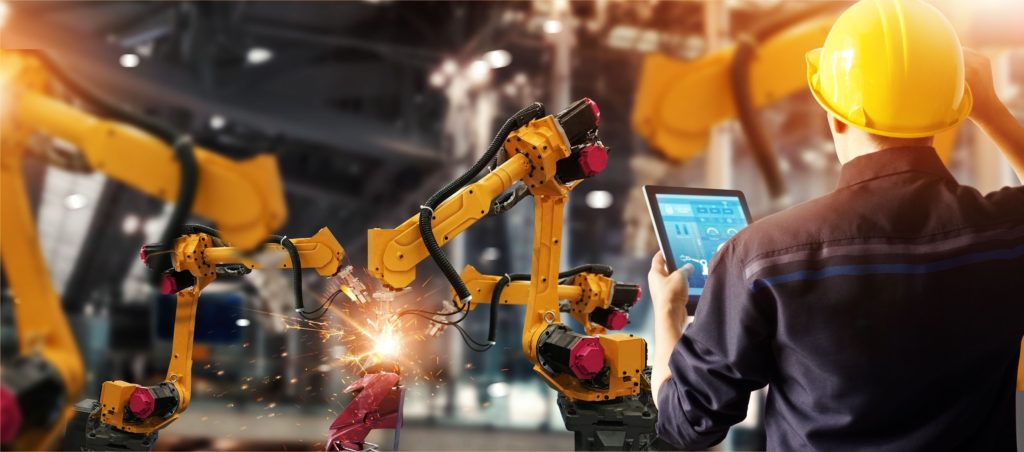
At a time with historic wealth inequality and an almost unprecedented unemployment rate, this may strike to the heart of the concerns shared by many who seem uncomfortable with technological progress. This problem threatens not only economic safety but also the mental health and daily stability of those left unemployed. People will need to figure out how to survive these losses economically but also psychologically.
It’s still likely, according to some sources, that technology will create more jobs than it replaces. However, those who are unable or unprepared for the new positions are left wondering what they will do during such a transition.
Bias in Data:
While risks to privacy and job security—such as those we’ve discussed—are pressing, there is an even more alarming layer of risks to AI systems we have not yet touched on. We discussed algorithms in part one of this series. We explored how AI systems are trained on big databases of information: creating their own unique algorithms until they can reliably provide the expected output. But what happens to the algorithm, and the system, when the data is biased, incomplete or contains other types of errors?
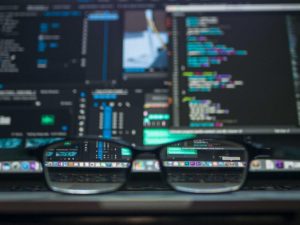
Biased data creates biased algorithms. A straightforward example of this risk are image datasets, which are widely used in training a variety of AI systems on image recognition. A Frontline report from 2019 found that image recognition software makes a variety of common errors based on racial and gender bias in image databases. For example, men in the kitchen were tagged as women a large percentage of the time.
One of the more concerning examples of these biased algorithms is in the criminal justice system. Some locations have adopted AI systems to help determine criminal sentencing by predicting whether a criminal is likely to reoffend or poses a significant risk to the community. ProPublica did an in-depth investigation of these systems and found that their recommendations are significantly racially biased.
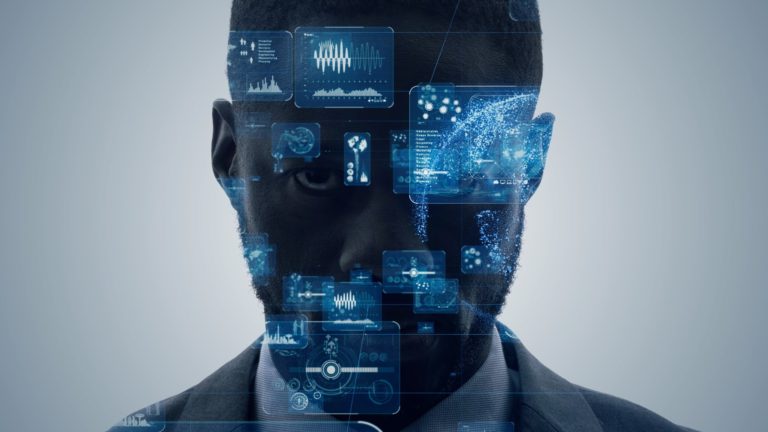
Because American law enforcement and incarceration have long been biased, the data that comes out of these systems is biased. The result is that any AI trained using this data comes to be biased in its predictions, which then reinforces the system’s bias.
Equality among the races, sexes and classes has not yet become a reality, so the information that comes out of our societies is also not equal. When datasets are used and designers haven’t actively considered and taken account for these sorts of concerns, the bias in the data becomes the bias in the new systems.
This problem is compounded because many companies refuse to make the algorithms public, considering them proprietary information. This means that the systems could be using any information provided in their calculations, including but not limited to: gender, race, zip code, credit score, sexual orientation, family, professional associates or even medical history.
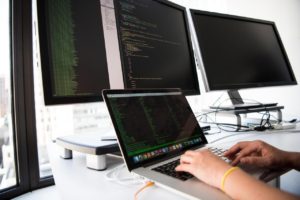
Strategies for positively integrating technology:
Ok, so what do we do about all of these risks? There are a variety of strategies that could help to make a difference in how things progress. While they will all require planning and effort to implement, the long-term social value would be well worth it.
- First, we need to acknowledge that data is now valuable. Privacy is often violated by companies looking to use our data to increase their bottom lines. If your data has so much value, it shouldn’t be freely available for commercial purposes.
Several US states and the European Union have instituted policies about data collection which are designed to protect consumers. Many locations now require websites and online services to offer full disclosure about any tracking that they do, and users can opt out of having their information collected. Expansion of these policies are not popular with all businesses but consumers are winning the right not to provide this free service to the company.
- The next strategy is closely related to the first in some ways. It is the idea that our economies and companies need to change and adapt to the current reality. This can mean a lot of different things—depending on who you ask. There are minor changes, such as allowing consumers to opt out of data collection, as we just discussed. Even small efforts can help to provide consumers with some measure of personal protection.
Other more significant systemic changes, such as universal basic income, have been suggested by some and may prove necessary. If companies focus on educating employees and integrating technology, rather than just saving money by replacing employees, some studies suggest automation could result in higher productivity and long-term increases in the number of available positions.
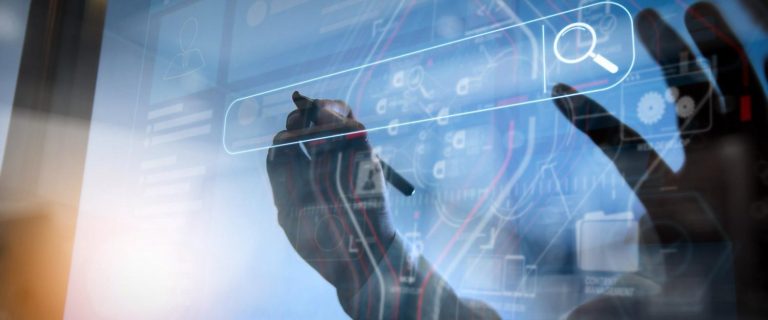
- One highly important strategy is to be conscious and responsible for protecting our own data privacy. There are various tools to secure your devices and data and protect your digital privacy and security. These can include things like using a virtual private network (VPN), secure and private passwords, updating your privacy settings for apps and websites, and keeping all software and operating systems up to date. Many services also now offer credit report monitoring for early warnings related to potential identity theft and similar crimes.
- Perhaps the crucial strategy of managing the risk of biased data are well-trained and conscientious data scientists. Data scientists seek out, prepare and use AI systems to analyze data, looking for specific types of information. For example, a data scientist may be employed to create a system to help select job candidates. To do this, the scientist must collect all the available data about the company’s current and past employees. Then, they must clean up this data. This generally means formatting the data but it should also include some assessment of the data and its implications.
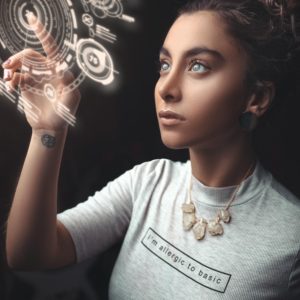
If the data identifies that female employees are paid substantially less than their male colleagues, then the data scientist should be mindful of this information. It may make a significant difference if, for example, women tend to quit working for the company after shorter employment periods than male employees. This data doesn’t mean that female employees are going to be less valuable to the company in the long-term, particularly if the problem of bias within the company is addressed; however, if the scientist doesn’t identify and control for this discrepancy in the data, the algorithm will be more likely to recommend male candidates.
Data scientists can make a world of difference in how accurate and universal a system has the power to be. Without their oversight, the data’s bias can seep into systems and predictions, potentially affecting our everyday lives, from credit applications to job applications. Ensuring that all data scientists are given an understanding of how important the human element is to their work could be a highly effective strategy.
- The final (and most crucial) element that will matter in how we manage these issues is awareness. All of the strategies we discussed require that people—from academics and politicians to individual citizens—not only be aware of the issues but to discuss solutions. We need people to consider, discuss and guide the course of these changes because silence will result in abandoning progress to fate.
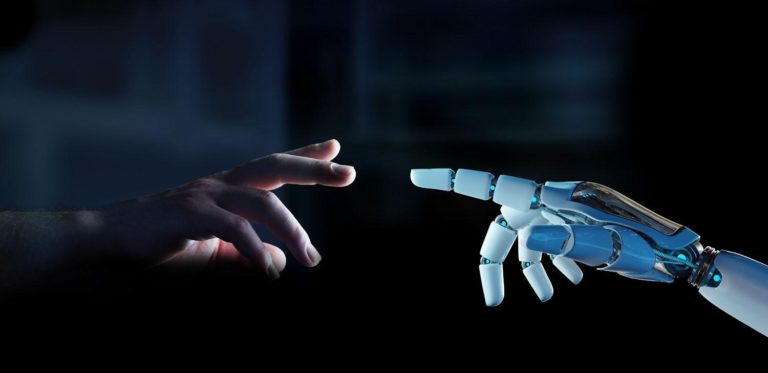
There are no simple solutions, but now is the time to address these issues. Technology and AI will continue to develop and will reach heights that seem unimaginable to us—even now. Over time, datasets and algorithms used in one system may be recycled and reused in future applications. Much like building a skyscraper, if the foundation is not level and well-constructed, upper levels of the project will become unstable.
Because our AI technology is not very advanced yet, the risks of a robot or Roomba uprising is incredibly remote. That doesn’t mean the dangers posed by AI technology are not real. The risk posed by the advancement of AI has the potential to alter society on a fundamental level. The progress of AI will shape our lives and communities in countless ways, so let’s begin by engaging in the conversation and each taking part in deciding the direction of progress.